Using Machine Learning with Physical Models to Predict Extreme Events
-
-
Department of Mechanical Engineering
Filed Under
Recommended
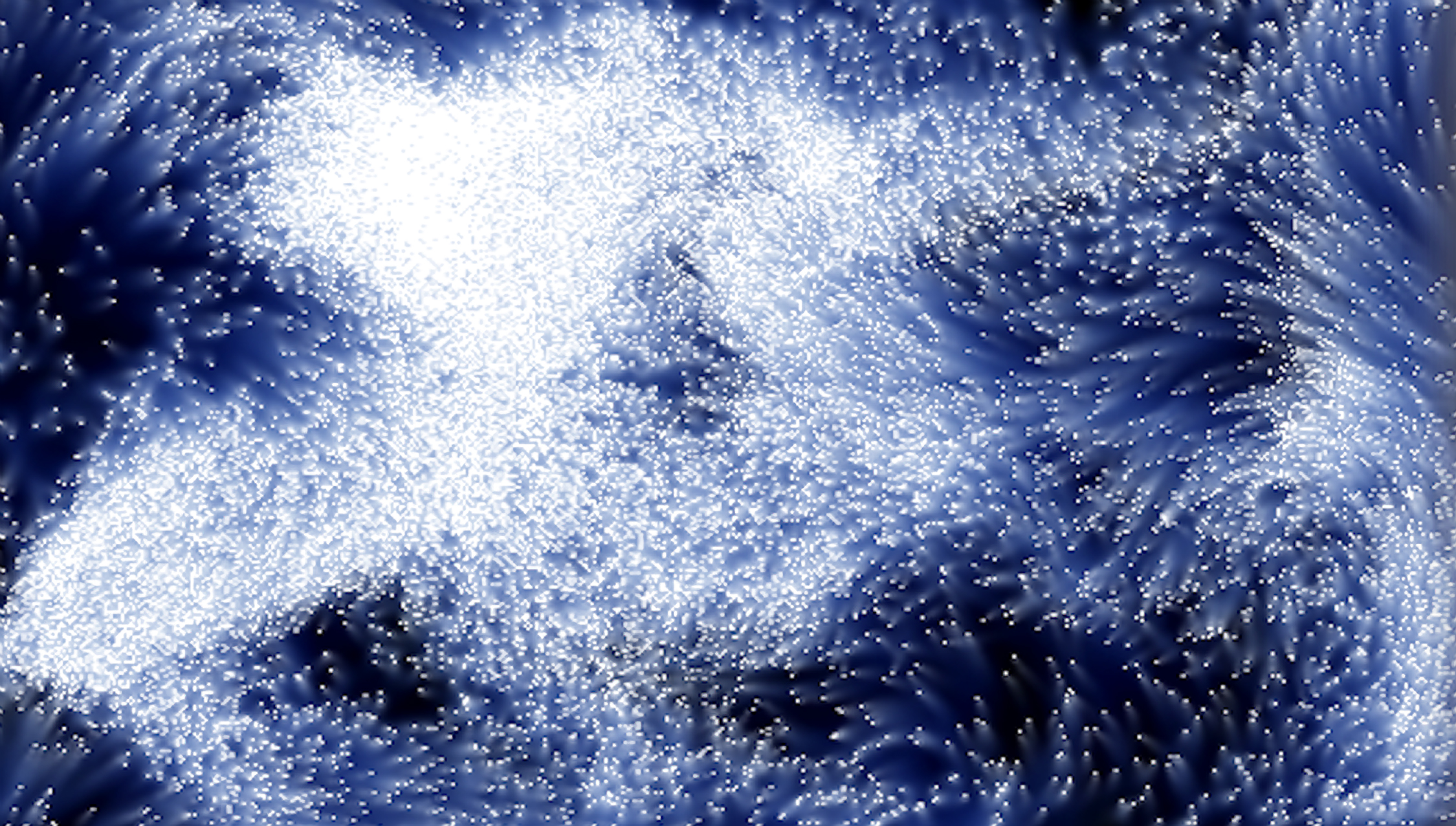
2017 was one of the most destructive hurricane seasons on record. Hurricane Harvey left the streets of Houston flooded. Hurricane Maria ravaged Puerto Rico, leaving millions without power for months. Hurricane Irma decimated the infrastructure of many Caribbean Islands.
The intensifying power and increasing unpredictability of Atlantic hurricanes have left meteorologists searching for ways to make more accurate forecasts and give people enough warning to prepare or evacuate. Themistoklis (Themis) Sapsis PhD '11, associate professor of mechanical and ocean engineering and principal investigator at MIT’s Stochastic Analysis and Nonlinear Dynamics (SAND) lab is hoping to improve our ability to predict extreme events like hurricanes. By using machine learning to enhance existing models, Sapsis is training computers to make more accurate and reliable predictions. He explains in a Q&A below.
Associate Professor Themis Sapsis and his team aim to predict the motion properties of finite-size particulates moving in arbitrary fluid flows — such as bubbles in water (see photo above).
Associate Professor Themis Sapsis
You began your academic career with a degree in naval architecture. How did you transition from naval architecture to the prediction extreme weather events?
I was always inclined toward mathematics so all of my research has been math oriented with applications in ocean engineering. After completing my PhD in mechanical engineering at MIT, I had a post-doc appointment at NYU’s Courant Institute of Mathematical Sciences. I then came back to MIT as a faculty member with the idea of solving problems in ocean engineering by developing or utilizing new mathematical methods
One area my lab and I study is the general prediction of extreme events. We have done extensive work on the prediction of extreme water waves – also known as rogue waves – in the ocean, but we are also interested in extreme events in engineering and geophysical fluid flows. For engineering applications, we want to be able to predict when an extreme event will occur so we can apply control strategies that can suppress or avoid the event. I’m trying to push the envelope by taking math and ocean engineering into the machine learning sphere.
Why are current models for predicting extreme weather events like hurricanes not fully reliable?
There are already comprehensive models in place that people have been using to predict weather events like hurricanes and nor’easters. Our aim is to build a mathematical foundation that combines available data with these physical models more effectively so that predictions are more accurate when it comes to extreme events. The challenge is that for extreme realizations we do not have enough data available to train the models. Therefore, it is even more essential to build effective blending strategies between models and data. Another important challenge is the computational cost associated with the comprehensive weather prediction models that we hope to reduce through these blended strategies.
Read the full Q&A on the MECHE website.