Using Data from Fictional Patients to Enable Real Covid Breakthroughs
-
-
Slice of MIT
Filed Under
Recommended
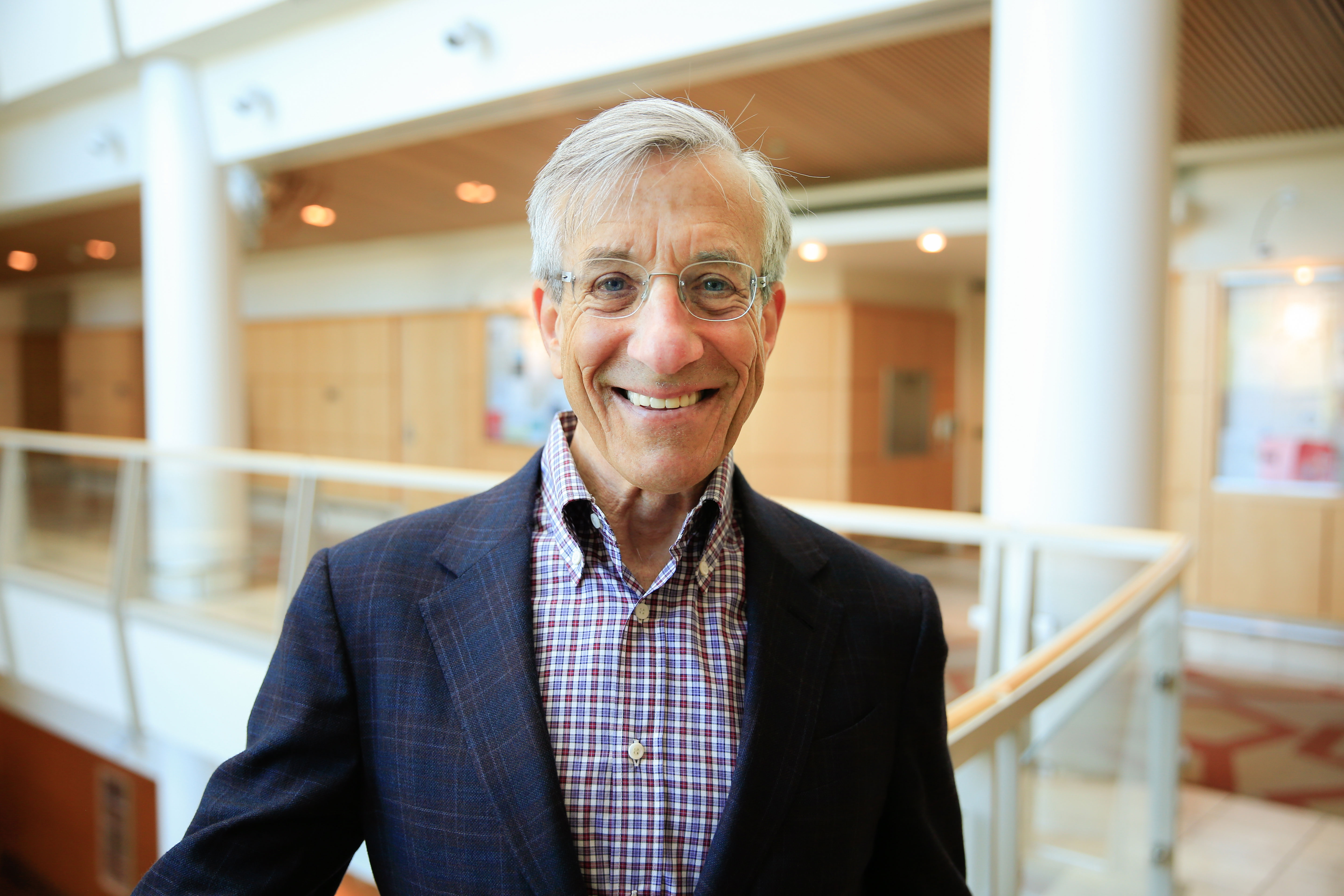
As the coronavirus pandemic moves through its second year, medical researchers face a dilemma. Millions of people in the US have contracted Covid-19, generating a vast trove of electronic medical records containing useful data for studying the disease. However, privacy regulations make it difficult to get access to that data quickly. So which matters more: faster research or reliable privacy?
Syntegra, a data-science startup cofounded by Michael Lesh ’77, SM ’77, aims to enable both at once. The company uses artificial intelligence software to create synthetic medical data with the same statistical properties as real-world medical records, but with personally identifying information irreversibly removed. Think of it as generating a parallel universe of Covid-19 patients: “They” don’t actually exist, so there are no privacy concerns. But the patterns present in their medical data—like the relationship between age, preexisting conditions, and severity of Covid-19 symptoms—are mathematically identical to the patterns that exist in the records of millions of real-world patients.
“Synthetic data makes this information immediately available in a way that the real data isn’t—or may never be—because of privacy concerns, which are important and valid,” Lesh explains. “The synthetic version maintains absolute privacy. You can’t ever work back to the real patients.”
In January 2021, Syntegra partnered with the National Institutes of Health and the Bill and Melinda Gates Foundation to create and validate a synthetic version of the country’s largest pool of Covid-19 medical records, comprising at that time more than 2.7 million patients and 413,000 positive cases of the disease. The goal, says Lesh, is to provide a mathematical guarantee that this parallel universe is an accurate, but completely anonymous, replica of the real-world records. With that guarantee in hand, “any researcher can apply for rapid access with minimal administrative friction,” Lesh says. “They would be able to immediately start doing research projects.”
Synthetic data makes this information immediately available in a way that the real data isn’t—or may never be—because of privacy concerns.
“I feel a company coming on”
Lesh has been building bridges between medicine and technology for four decades. He earned bachelor’s and master’s degrees from MIT in 1977 in computer science, electrical engineering, and biomedical engineering. “MIT is not just about facts and formulas. It teaches an approach to problem solving you don’t find anyplace else,” he says. The research for his master’s thesis was in collaboration with Dr. Sheldon Simon of Boston Children’s Hospital, where he used computers to analyze the muscle movements of cerebral palsy patients. “I saw how engineering was being applied to health care—we were actually helping kids walk better,” he recalls. “But I felt like I was developing solutions to problems that were being identified by others in closer contact with the patients. I wanted to identify the clinical need directly, not just engineer solutions after the need was already well defined. So I applied to medical school.”
After medical school and residency at the University of California San Francisco (UCSF), Lesh trained in cardiovascular disease and cardiac electrophysiology at the Hospital of the University of Pennsylvania. Lesh joined the faculty at UCSF in 1989, eventually taking the role of chief of cardiac electrophysiology. Lesh also used his MIT computer science background to develop a detailed model of arrhythmias, which led to a new understanding of atrial fibrillation (AF), the most common cause of stroke. He hypothesized that a new kind of minimally invasive treatment was possible using catheters, and filed several patent applications. “I was reluctant to license these AF inventions to a big company, because there was no guarantee they were going to be developed,” he says, “and I wanted to have this new catheter to treat the patients I was seeing every day.” So he started his own company to bring his catheter treatment to market.
That company, Atrionix, was acquired by Johnson and Johnson in less than three years. Between 1998 and 2011, he founded and served as CEO of four more successful medical-technology startups. “‘Physician-entrepreneur’ was an oxymoron at the time I started Atrionix. But as my wife will tell you, I’ll just become obsessed with a problem, the hair on the back of my neck will stand up, and I’ll tell her, ‘I feel a company coming on,’” Lesh says. Finding “unmet needs” at the bedside was always his north star. “I work with a lot of entrepreneurs, and often they have a technology that’s like ‘a hammer in search of a nail.’ My advice is to first find the nail.”
I work with a lot of entrepreneurs, and often they have a technology that’s like ‘a hammer in search of a nail.’ My advice is to first find the nail.
Synthetic data meets the pandemic
After his fourth startup was acquired in 2017, “I wasn’t planning on starting any more companies,” Lesh says. He became UCSF’s executive director of health technology innovation to help other physicians become entrepreneurs. One of his first projects was shepherding a collaboration with Google to develop machine learning software that could predict medical outcomes using data from UCSF patient records. “The idea was the data would be ‘de-identified’ using standard methods, and then Google would run their AI magic,” he says. “But it fell through because we could not guarantee a level of privacy that would make the compliance and legal administrators feel comfortable, even though the data would be de-identified.”
Lesh sensed another unmet need. The problem wasn’t just confined to UCSF’s unsuccessful Google collaboration—it was widespread. “It’s very hard to share real-world clinical data, because it’s siloed throughout the health care system to protect patient privacy. But to fuel breakthroughs in precision medicine, or to develop evidence-based new treatments, we need to share real-world data with the life science industry,” he explains. Maintaining privacy is an ethical mandate. But simple methods of de-identifying patient data—that is, scrubbing it of obvious information like names and addresses—would still leave it vulnerable to so-called linkage attacks, in which an anonymized dataset is cross-referenced against another dataset containing identifying information. (The concept was first demonstrated in 1997, when then MIT graduate student Latanya Sweeney SM ’97, PhD ’01—now a computer scientist and privacy expert at Harvard—accessed the Massachusetts governor’s personal health records by matching supposedly anonymized hospital data against an electoral roll database she purchased for $20.)
Lesh investigated more rigorous mathematical techniques for ensuring privacy, but they all had “significant limitations,” he says. Then he learned about synthetic data. “I learned all I could about it, and even found that it had been applied to medical records before,” he recalls, “but not in a comprehensive way and not very accurately.” His nail now had a hammer. He could feel a company coming on. In 2019, Syntegra was born.
Syntegra uses an AI system called GPT-2, which made headlines in 2019 for its ability to generate fake-but-convincing newspaper articles after being trained on real-world samples. Lesh and his cofounder, Ofer Mendelevitch, realized that GPT-2 could use the text from real health records to create synthetic look-alike patients, whose information could then be given to researchers with no risk of exposing the real patients. (Syntegra performs extensive tests to show that the statistical properties of its synthetic data precisely match those of the original health records.) Lesh and Mendelevitch raised $3.1M in seed financing in early 2020.
The new startup was soon approached by the Bill and Melinda Gates Foundation’s data science group, which wanted to use synthetic data to democratize the results of clinical trials it had funded to improve health in the developing world. “Researchers at the foundation believe that the best science will come from multiple researchers having access to a lot of data. Not only is privacy an issue, but each country has its own regulations, so researchers could not combine the clinical trial data from multiple countries,” Lesh says. “We had a number of planning sessions on using Syntegra’s synthetic data engine to combine all this data and make it widely accessible. And then Covid hit.” The foundation, Syntegra, and the NIH Covid coalition, N3C, teamed up to validate the fidelity and privacy of synthetic Covid data.
“Covid is not going away, and there’s a lot we still don’t know about this disease,” Lesh says. Making synthetic patient-level data quickly and safely accessible, he explains, will speed up scientists’ efforts to understand the disease and develop new treatments. “Randomized clinical trials are important,” Lesh explains, “but they are expensive, take time, and the data is not publicly available. Synthetic data, meanwhile, can be shared with scientists immediately while maintaining total patient privacy.” Predictive models—like determining the chance that a Covid patient will need to be placed on a ventilator—can be developed with the synthetic data and then applied to real patients more quickly.
“One of the biggest hurdles in the advancement of clinical science is data availability. AI-enabled synthetic data generation is a unique engineering approach to that problem,” Lesh sums up. “If this method is further validated, it could significantly accelerate bringing new treatments to patients.”
Portrait of Michael Lesh (top) by Sean David Karlin.