Alum Joins MIT Faculty to Bond Computing with Chemistry
-
-
MIT Spectrum
Filed Under
Recommended
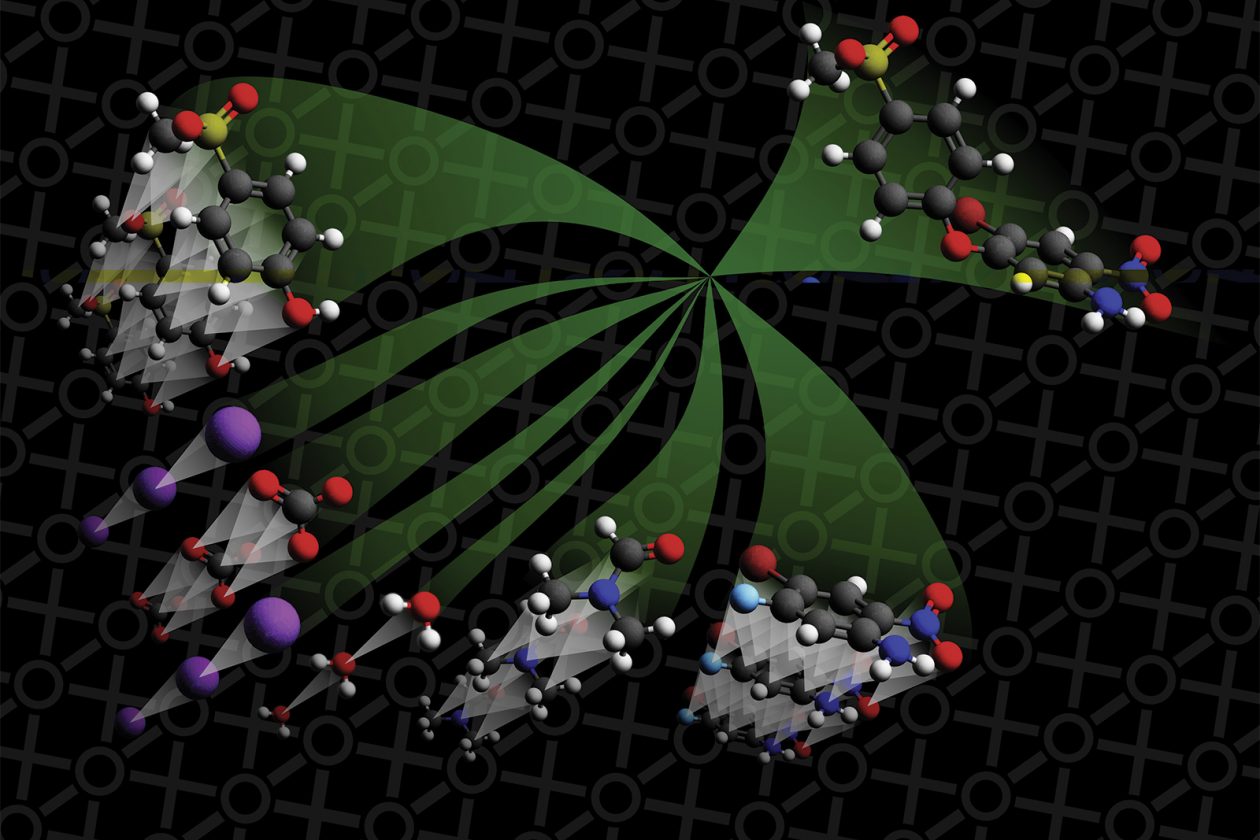
Chemistry and computer science may not seem like the most obvious pairing: one conjures the image of a lab-coated and begoggled scientist titrating agents in test tubes and beakers, while the other brings to mind a scientist hunched over a computer, typing code and analyzing vast data sets. And yet, Connor Coley SM ’16, PhD ’19 is building his career at the interface of these fields, developing algorithms and machine-learning systems to streamline the work chemical engineers do in the lab—tools he hopes can accelerate the process of discovering and synthesizing useful molecules.
“I would consider myself very application-driven,” says Coley, who was named to Forbes’s 30 Under 30 health care innovators in 2019. “I want to work on problems where I can improve the way that other people are able to approach their own research.”
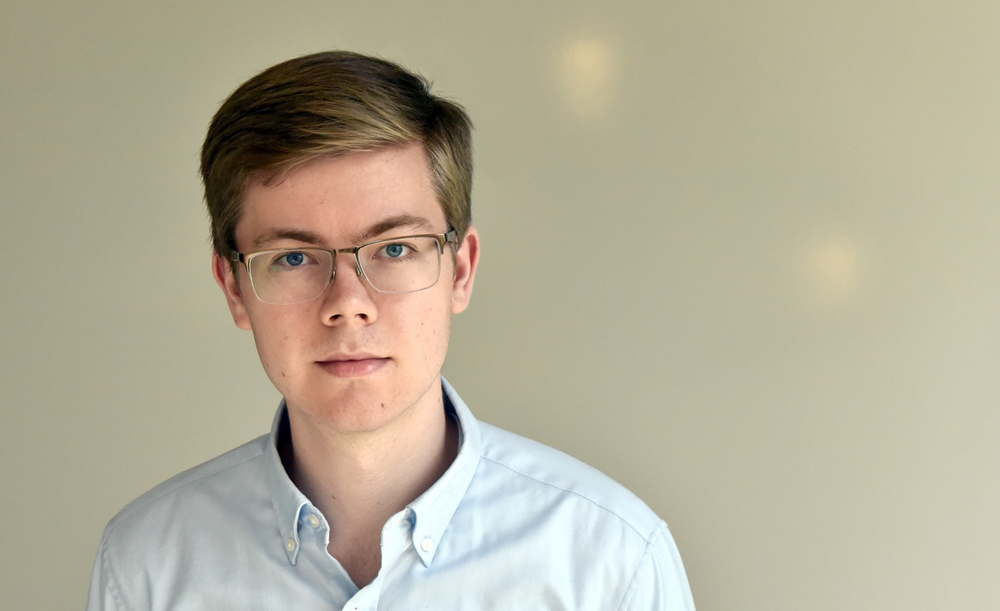
While Coley has always enjoyed coding and programming, he considers these interests secondary to his passion for chemical engineering—his undergraduate major and the focus of his master’s degree. It wasn’t until Coley started working on his PhD in chemical engineering, supervised by Klavs Jensen, the Warren K. Lewis Professor of Chemical Engineering, and William Green, the Hoyt C. Hottel Professor of Chemical Engineering, that he thought to combine his interests.
Coley was in the lab, building automated reaction platforms that use algorithms to optimize conditions for existing chemical reactions, when he realized that another part of the process could be made more efficient: designing the reactions themselves.
“Once you’ve figured out what molecular structure you want to make, you still need to come up with a recipe—all the ingredients, all the instructions, all the steps that it will actually take to physically make it,” Coley explains. This process requires chemical engineers to draw on published papers, previous experiments, and general chemistry knowledge. “My interest was trying to use that background information in a more principled way.”
Once you’ve figured out what molecular structure you want to make, you still need to come up with a recipe—all the ingredients, all the instructions, all the steps that it will actually take to physically make it.
Working with group members, Coley has built an algorithm-based, machine-learning system, trained on millions of previously published reactions, that analyzes this background information and offers chemists options and suggestions for making molecules. “It’s a way to supplement, not replace, the more traditional approaches,” Coley says.
The research has been published in Science, and an open-source version of the system is available through MIT’s Machine Learning for Pharmaceutical Discovery and Synthesis Consortium. This version has been adopted by chemists and chemical engineers in industry and academia, many of whom conduct pharmaceutical research. “A lot of the molecules that we think about are or one day could be drugs,” Coley says.
A lot of the molecules that we think about are or one day could be drugs.
As a postdoctoral researcher at the Broad Institute of MIT and Harvard, Coley temporarily shifted his focus to molecule discovery using a technology called DNA-encoded libraries. In this approach, Coley explains, chemists put millions of DNA-tagged compounds in a tube and simultaneously screen those compounds to see which ones have the greatest affinity for a target—for example, a protein linked to a disease. A selection process then identifies the molecules most inclined to “stick” to the target, measured through DNA amplification and sequencing. Chemists typically look only at measurements related to those top molecules, ignoring the rest.
Coley wants to improve this process by developing computational tools that can sift through the entire collection of measurements and pull out anything that may improve the design of molecules selected for development. “If we have a better understanding of all the different molecular structures that correlate with affinity to our protein, it will be easier for us to tweak the other properties that matter,” Coley says.
Whatever the future brings, Coley knows his next step: as of July 2020, he is an assistant professor in the Department of Chemical Engineering. “MIT is a very fun ecosystem to be a part of,” a place that recognizes the value of applied research and interdisciplinary collaboration, Coley says.
Ultimately, Coley hopes his work will improve the research process for thousands of scientists—making all of their discoveries and advancements a little bit faster. “That can have a pretty sizeable impact."
This story originally appeared in the Spring 2020 "Computing" issue of MIT Spectrum magazine.
Illustration (top): molecules at bottom left move through a neural network model (center). The single compound at the top right represents the product that the model believes will be formed. Credit: Connor Coley