Revolutionizing AI by Reproducing the Neural Circuitry of the Zebra Finch
-
-
MIT Spectrum
Filed Under
Recommended
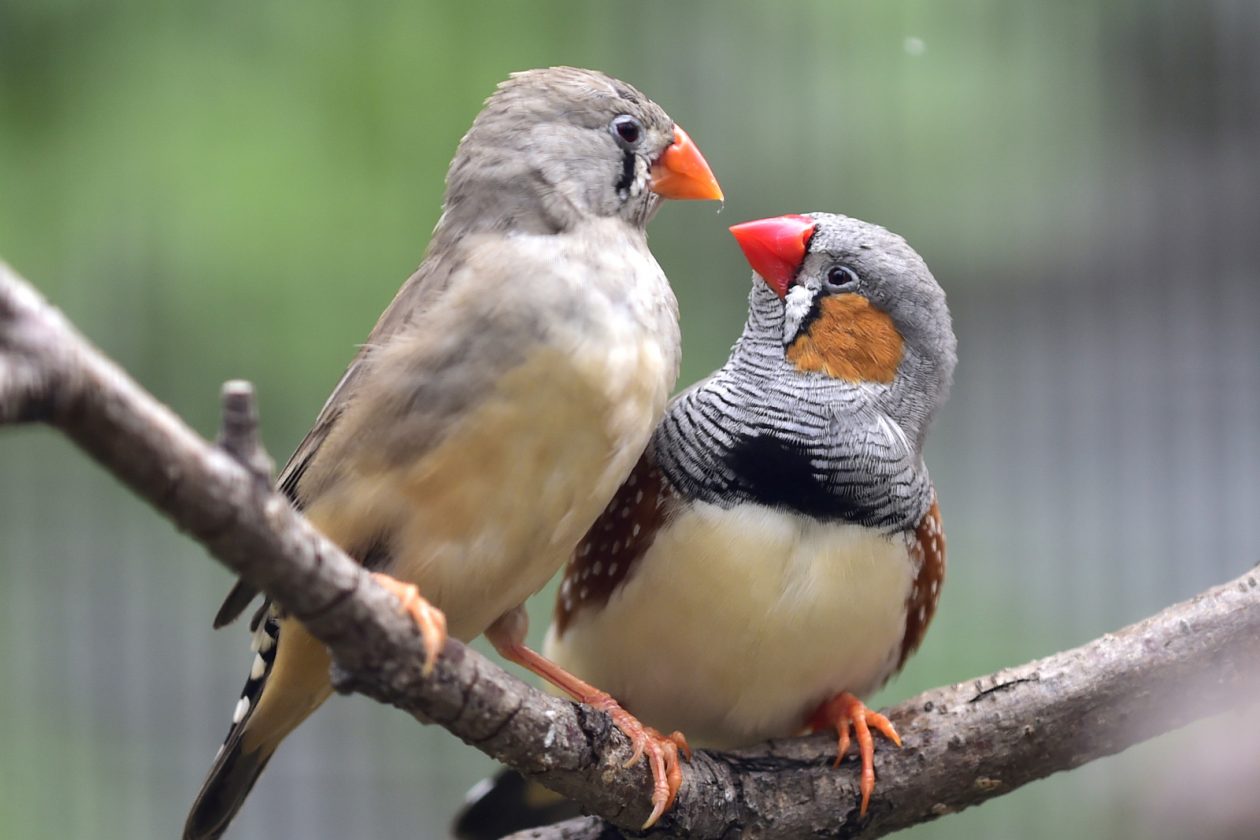
One month after being hatched, male zebra finches start learning to sing by imitating the songs of their fathers. Practicing thousands of times a day, young finches master these songs in a few months so they can eventually pass on the classics—sometimes used in courting rituals—to the next generation.
For decades, scientists have recognized that the songbird learning process could shed light on how humans acquire languages and other skills. Now, a team of MIT researchers is going a step further: in a novel collaboration supported by the MIT Quest for Intelligence, they are trying to revolutionize artificial intelligence (AI) technology by reproducing, in the form of computational hardware, a vital component of the zebra finch’s neural circuitry.
The lead investigators—Jesús del Alamo, the Donner Professor and professor of electrical engineering and computer science; Michale Fee, head of the Department of Brain and Cognitive Sciences and the Glen V. and Phyllis F. Dorflinger Professor; Ju Li PhD ’00, the Battelle Energy Alliance Professor of Nuclear Science and Engineering and Professor of Materials Science and Engineering; and Bilge Yildiz PhD ’03, the Breene M. Kerr Professor of Nuclear Science and Engineering and of Materials Science and Engineering—bring to this project a broad range of expertise.
Fee, who is also affiliated with the McGovern Institute for Brain Research, has studied songbird learning from a basic science perspective since 1996, though he hadn’t focused on applications of his research until joining forces with the others in 2021. In a 2020 paper for Nature Communications, del Alamo, Li, and Yildiz reported on their artificial synapse device, which incorporated new materials and a new electrochemistry-based approach to emulate biological synapses, or connections between neurons. This approach yields low-energy consumption that is approximately one million-fold lower than conventional silicon-based technology and close to those of biological synapses.
“Many new avenues are emerging for AI technologies, and almost all of them will require new materials and new system architectures,” del Alamo says.
The latter research had nothing to do with zebra finches, but Yildiz remembered an intriguing talk given by Fee in 2019 and approached him about collaborating. “We found that there was indeed a good opportunity here,” she says—one that Fee was eager to pursue.
“When a bird learns its song, it tries out different things,” he explains. “Sometimes that makes the song better; other times it makes it worse.” He identified a crucial bit of circuitry in the finch brain, showing that synapses are strengthened when three elements are aligned: the context (where the bird is in a given song), action (a change introduced at that juncture), and reward (the benefit of an improved outcome).
In a real biological neural network, synaptic strength is controlled locally rather than centrally, Fee adds. “Each synapse learns to adjust itself.”
Electrochemical Control
Now del Alamo, Li, and Yildiz are building a new kind of neural network based on Fee’s learning model. Rather than relying on the energy-intensive transistors used in standard neural networks, they are controlling synaptic strength the way the brain does it: electrochemically shuffling ions. This means regulating the flow of electrons and positively charged ions into and out of the artificial synapses. Li and Yildiz are still working out the precise blend of materials and electrochemistry of their reconfigured network, while del Alamo is shrinking down the size of the electrochemical synapses to nanoscale dimensions.
The implications of this work extend far beyond a single device built around principles of songbird learning, del Alamo asserts. “Many new avenues are emerging for AI technologies, and almost all of them will require new materials and new system architectures that are much more energy-efficient than present approaches.”
The current effort is already providing clues as to what future computer architectures might look like, but it may also help illuminate key questions in neuroscience, Yildiz says. “The brain is a closed box, but the system we’re developing could be an open box to illustrate and test how learning works.”
Steve Nadis is a 1997–98 MIT Knight Science Journalism Fellow.
This story originally appeared in the Fall 2021 issue of Spectrum.
Photo: Wang Liqiang.